Information Technology Reference
In-Depth Information
Fig. 7.2 Average error ratios
in the classification between
class 1 and class 2 for
different values of the class
persistence parameter a
;
corresponding to SICAMM
(solid line) and ICAMM
(dotted line)
Error ratio
0.2
0.18
0.16
0.14
0.12
0.1
0.08
0.06
0.04
0.02
0
0.5
0.55
0.6
0.65
0.7
0.75
0.8
0.85
0.9
0.95
1
α
Note that for a [ 0
:
6 the error percentage of classification starts to decrease
when using SICAMM, while it remains constant when using ICAMM. This is an
obvious consequence of the capability of SICAMM to exploit the sequential
dependence of successive classes.
7.1.4 Analysis of Hypnograms
In this section, we show a practical application of SICAMM to a real data problem.
The framework is computer-assisted sleep staging [
10
]. Typically, human sleep is
divided into four different stages: awake, light sleep, deep sleep, and rapid-eye
movement (REM) sleep. Determining the stage of a patient over a long period is
relevant to the diagnosis of different sleep disorders and other human illnesses.
The sequential record of the different sleep stages corresponding to a given period
is called a hypnogram. Hypnograms of a patient are usually obtained in an non-
automatic manner by experts making a visual inspection of the so-called poly-
somnograms (PSG), which is a set of EEGs and other records obtained from the
patient while sleeping. Some degree of automaticity has been implemented to help
the experts [
10
], but a totally automatic system is still a challenge.
Of particular interest is the detection of very short periods of wakefulness [
11
]
(also called arousals) since their frequency of appearance are related to the pres-
ence of apnea and epilepsy. Thus, we have applied ICAMM and SICAMM to
obtain the automatic detection of arousals. Hence, the hypnograms will show only
two stages: ''stage 1'', which corresponds to sleep (in any of the possible sleep
stages); and ''stage 2'', which corresponds to arousal. The results obtained from
ICAMM and SICAMM are compared with the non-automatic detection made by
an expert.
The observation vector, which is the input to the classifier, has four feature
elements. Every feature is computed in very short segments of the PSG signals
(typically 1-3 s) and averaged in epochs of 30 s. Then, a decision about the sleep
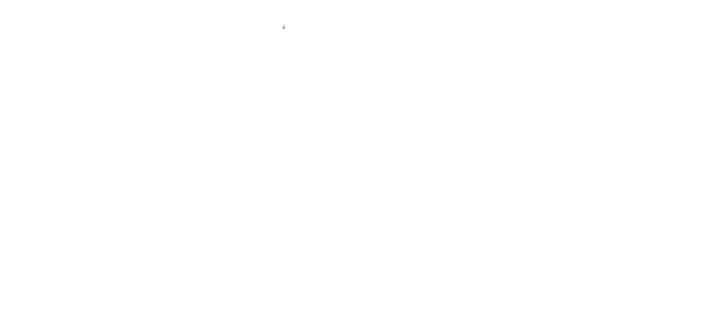


















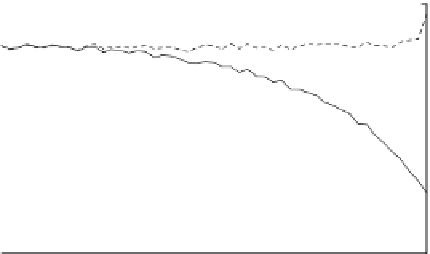




























Search WWH ::

Custom Search