Chemistry Reference
In-Depth Information
1.40
1.20
1.00
0.80
0.60
0.40
0.20
-0.06
-0.04
-0.02
0.00
0.02
0.04
0.06
0.08
0.10
0.12
0.14
[Effect]
FIgure 5.4
Example probability plot. In this example, the factor effects are plotted against
a linear distribution; departures from the line would indicate robustness issues.
features can be used not just to generate the data, but also to analyze and report the
data during method validation.
While a comprehensive statistical discussion is outside the scope of this chapter,
several good references are available for more detail [5-7]. Essentially, the analyst
must compare the design results, or the results obtained from the experiments run,
for example, according to Table 5.1, or Figure 5.3, to the nominal results. Regression
analysis and calculation of standard or relative error are common ways to look at the
data. Analysis of variance (ANOVA), which is a test that measures the difference
between the means of groups of data, is another common way of making the compar-
ison. Sometimes called an F-test, ANOVA is closely related to the t-test. The major
difference is that, where the t-test measures the difference between the means of two
groups, an ANOVA tests the difference between the means of two or more groups.
The primary goal of any robustness study is to identify the key variables or fac-
tors that influence the result or response, and graphs are an easy way to observe
the effects at a glance. Effects plots, or probability plots, are two common ways to
represent robustness data, and most general-purpose statistical software programs
can be used to generate probability plots. As illustrated in Figure 5.4, in a prob-
ability plot the data is plotted in such a way that the points should approximately
form a straight line. Departures from the straight line indicate deviations in the
data that affect robustness. Different types of probability plots, called normal or
half-normal probability plots, are used to further qualify the data. Normal prob-
ability plots are used to assess whether or not the data set is approximately nor-
mally distributed. Half-normal probability plots can identify the important factors
and interactions.
An effects plot is another type of graphical representation, as depicted in
Figure 5.5. Similar to a bar chart, or histogram, the effects plot can also identify the
important factors and interactions.
5.5 documentAtIon And reportIng
As the saying goes, if it isn't written down, or documented in a report, it never
happened. Proper documentation of the robustness study is, of course, essential
to the method validation process. A properly constructed report must include the
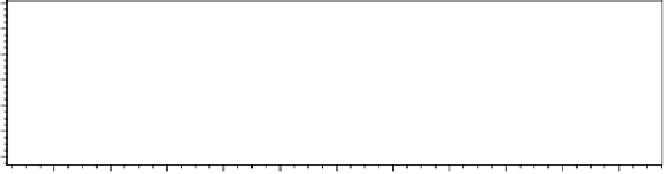







