Agriculture Reference
In-Depth Information
tions from the native range of RIFA in South America were extracted from pri-
mary literature [31-33] and georeferenced as latitude-longitude coordinates.
We used Forward-ENM to develop native-range ecological niche models to
predict the potential invaded distribution of RIFA in the US based on the 71
South American occurrence points, divided at random into near-equal sets of
training data (used to build models) and test data (used to assess accuracy).
Because the process of model development is stochastic, and resultant GARP
models vary in quality, we used a procedure described by Anderson et al. [34]
to select the best subset of models. In short, we generated (roughly 1,000)
Forward-ENM models until GARP produced 100 models with omission errors
of zero. We calculated the mean area predicted to have RIFA present from
these 100 zero-omission models; then from these 100 models, we selected the
ten models closest to this overall mean area. The ten best models were import-
ed into ArcGIS 8.3 and summed to assess the degree of model overlap, which
is a measure of model confidence. For example, regions where all ten models
predicted presence of RIFA had a value of 10, or maximum model agreement,
whereas regions where few models predicted presence of RIFA had low val-
ues, or minimum model agreement. The known ranges of RIFA, as determined
from published maps [21, 33], were overlaid on the summed predictions for
comparison.
Next, using Reverse-ENM and the 771 invaded US county center-points
(divided as before into training and test datasets), we developed invaded-range
ecological niche models to predict the potential native distribution of RIFA
within South America. To select the best subset of Reverse-ENM models, we
used a method similar to that employed for Forward-ENM; however, instead
of selecting the ten models closest to the overall mean area predicted to have
RIFA present, we selected the ten models with the
smallest
area predicted to
have RIFA present from 100 Reverse-ENM zero-omission models. By choos-
ing the models with the smallest “presence areas” we ensured, to the largest
degree possible, that differences between the Forward- and Reverse-ENM pre-
dictions were the result of actual differences in niche occupancy in the US and
South America, and not the result of commission errors. The ten smallest
Reverse-ENM models were imported into ArcGIS 8.3 and summed, and the
known ranges of RIFA were overlaid for comparison.
Results and discussion
The distribution predicted using Forward-ENM closely matched the observed
native range of RIFA along the northern, western and southern boundaries
(Fig. 2A). The western boundary of RIFA clearly abuts the Andes mountain
range, while the northern and southern boundaries appear to coincide with
transitional zones into the wetter climates of the Amazon basin to the north and
the colder and drier climates of central Argentina to the south. The model suf-
fered from commission error along its eastern boundary. Commission error is
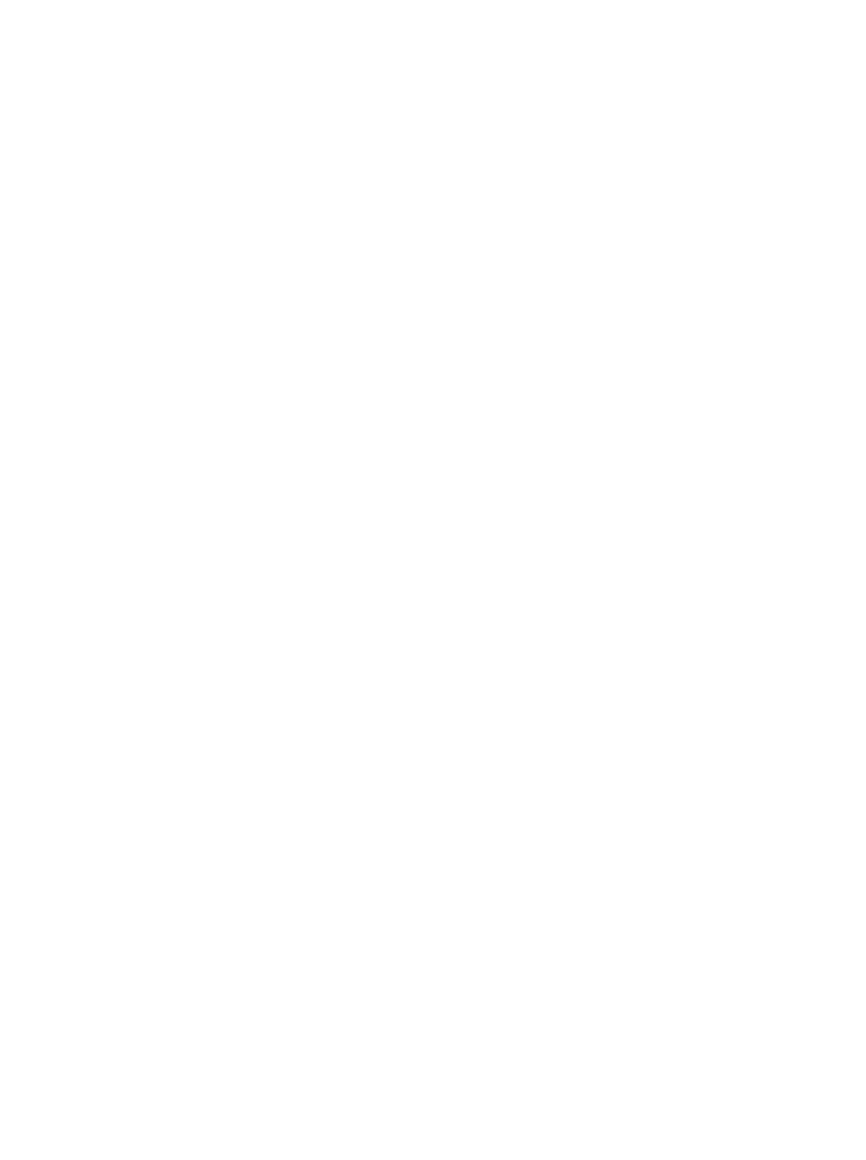
Search WWH ::

Custom Search