Information Technology Reference
In-Depth Information
Decentralized Reputation Mechanisms
The main problem with the centralized reputation mechanisms is that they are not suitable for
open Multi-Agent Systems given that the MAS nature is intrinsically not referred to a central
authority, but prevails in the distribution of the various attitudes to the agents who are possibly
considering the emergence of the global phenomenon.
An interesting mechanism, developed by Yu and Singh (Yu and Singh, 2003), take into
consideration witnesses as information sources. In this way the system (called
referral system
)
is based on the individual agents' knowledge and help, exploiting the agent's contacts for
each agent in the system. In addition, agents cooperate with each other with respect to these
referrals building in fact recommendations to contact other agents. Using referrals each agent
has to store a list of agents it knows (acquaintances) with their expertise and to contact them
in case of necessity. It is also possible that an agent who is unable to give answer to a query
gives back referrals pointing to agents it believes to have the desired information.
A mixed centralized/decentralized approach is introduced by (Jurca and Faltings, 2003).
They start from two main questions: Why should agents report reputation information? And,
why should they report it truthfully? They think about a set of broker agents, buying and
aggregating other agents' reports and selling back reputation information to the agents when
they need it. The proposed payment scheme guarantees that agents reporting incorrect or false
information will gradually lose money; on the contrary, the honest agents will not lose money.
A reputation model in which the trust evaluation process is completely decentralized has
been introduced by (Sabater and Sierra, 2001) and its name is
Regret
(Sabater, 2003). In this
model each agent can evaluate others' performance after each direct interaction has recorded its
ratings in a local database. In fact in
Regret
the agents can evaluate trust by themselves, without
any reference to a central mechanism. The Regret's authors called
direct trust
the trust value
derived from these ratings: They are calculated as the weighted means of all ratings (where
the weight depends from the recency). Similarly to
SPORAS
,
Regret
measures the predictive
power of each trust value through the two reliability measures: the number of ratings taken
into account in producing the value of trust and the deviation of these ratings.
In
Regret
the agents can also share their opinions. To this end the system develops a very
interesting and sophisticated witness reputation component (for aggregating witness reports).
This component strictly depends on the social network built up by each agent: in fact
Regret
uses social network to find witnesses, to select the witnesses for consulation and for weighting
the witnesses' opinions. Finally,
Regret
introduces the concepts of
neighbourhood reputation
and
system reputation
. With
neighbourhood reputation
is meant the reputation of the target's
neighbour agents: It is calculated by fuzzy rules. With
system reputation
is meant a mechanism
to assign default trust values to the target agent based on its social role in an interaction (e.g.
service provider, consumer).
Concluding
Regret
uses various sources of trust information, is decentralized, and as a
consequence, satisfies the requirements for modelling trust in multi-agent systems. Its main
limit is about the fact that it does not specify how the social networks are to be built.
Another interesting reputation model is
FIRE
(Huynh
et al.
, 2006). It is a decentralized
model designed for general applications based on a variety of trust sources. In particular, these
sources include:
direct experiences
(coming from the agent's interactions),
witness reports
(coming from third-party references),
role-based rules
(provided by end users encoding beliefs
about the environment), and
certified reports
(references obtained by the target agent).
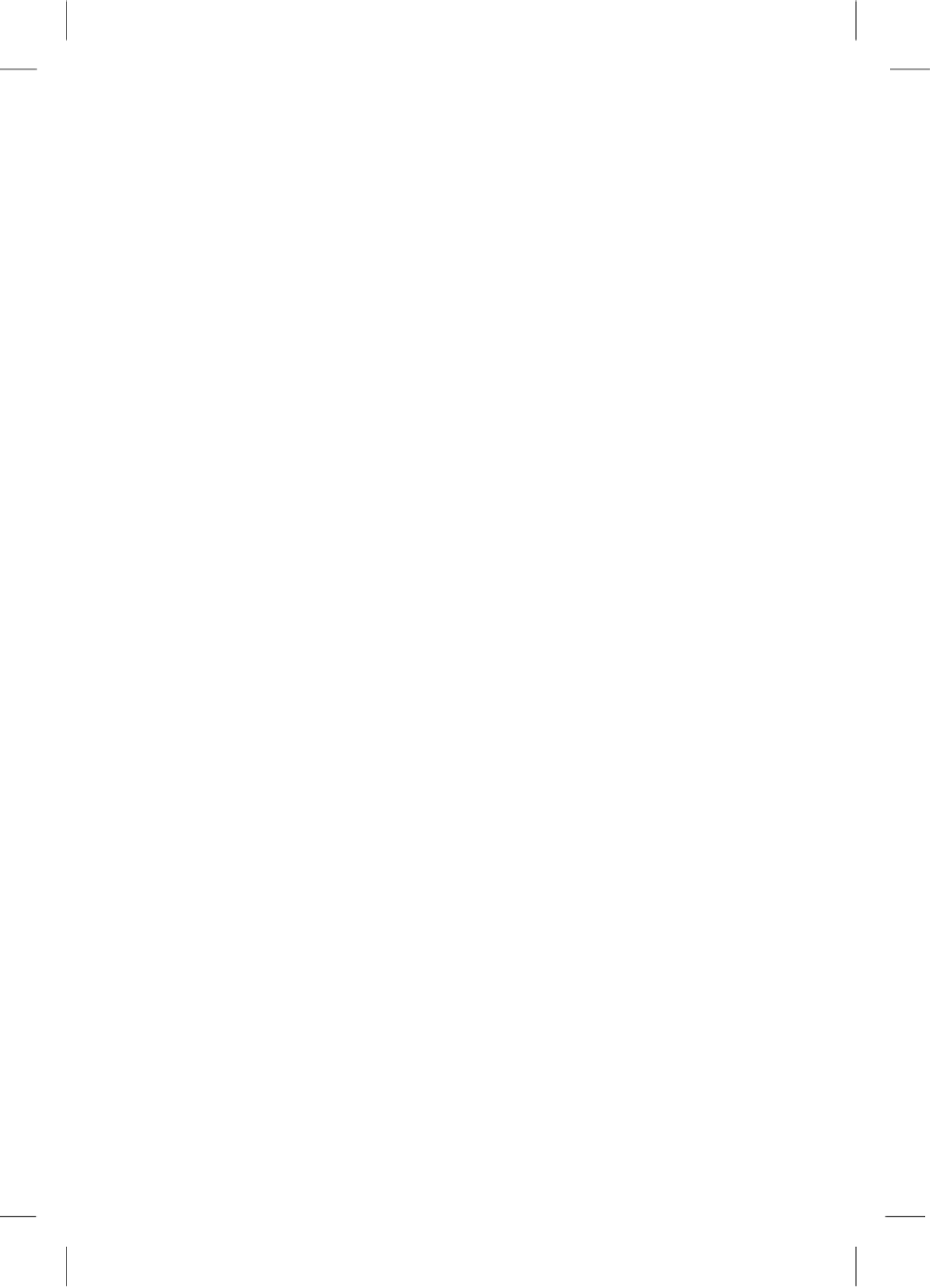
Search WWH ::

Custom Search