Information Technology Reference
In-Depth Information
The authors of the
KBA
(Kernel Boundary Alignment) aim at the potential prob-
lems in SVM like the border is slanted towards the minor-class, due to its lack of
representation in the dataset. In such case, they propose to modify the function of
nucleus adapting it to the distribution of classes and thus reshaping the boundary.
For KBA, the authors divided the dataset into 7 parts: 6 for training and 1 for test-
ing, and 7:3 in SDC case. The columns with the SVM and SMOTE methods are
included because they can be compared with the rest of the results (both authors
included them in their results). Both KBA and SDC are kernel methods, based on
modifications of a SVM.
The UCI datasets selected to do this comparison are: Segmentation, Glass, Car,
Yeast, Abalone and Sick. All of the selected UCI datasets do not have binary output,
and they were transformed into binary datasets by choosing one class as the negative
one and the rest of classes were grouped all as one. We chose the same class than the
authors of both methods just to compare the results.
In Table 4 the number that appears with the name of the dataset corresponds to the
chosen class. The first four columns of the table explains the type of the dataset,
the following three columns are the g-means results from the KBA and the next 3
columns the same results from the SDC. The last two columns reflect the g-means
results from the method proposed in this paper (FLAGID) and the different number of
rules of the systems found.
These results show that for the Glass, Segmentation and Yeast datasets our method
(FLAGID) is better, in terms of the g-means metric whilst for the Car and Abalone it
is worst. The results are equal for the Sick dataset. In case of Abalone FLAGID im
proves KBA but it is worst than SDC. Always the system found has a small number
of rules, i.e., so it has a high probability of being understandable by a human expert.
To prove that the method scales good when the imbalancing level increases, we
imbalanced even more some datasets. For instance, we imbalanced much more the
Table 2.4.
Comparison of our method (FLAGID) with the SVM, SMOTE, KBA & SDC
methods for some UCI datasets, by means of the g-means measurement. In first column, the
number of the class made as minor-class is included with the name of the dataset. The columns
2, 3 & 4 express the characteristics of every dataset. The last column indicates the number of
rules of the fuzzy system found, that shows that it is reduced.
SVM
(6:1)
SMOTE
(6:1)
KBA
(6:1)
SVM
(7:3)
SMOTE
(7:3)
SDC
(7:3)
FLA
GID #rules
Dataset
#attrib #pos #neg
Segmentation(1) 19
30 180 0.98 0.98
0.98 0.99 0.99
0.97 1
3,4,5,9
Glass(7)
10
29 185 0.89 0.91
0.93 0.86 0.87
0.94 0.97 3,6
Car(3)
6
69
1659 0.99 0.99
0.99
0
0.98
0.984 0.94 3,4
Yeast(5)
8
51
1433 0.59 0.69
0.82
0.83 3,6
Abalone(19)
8
32
4145 0.0
0.0
0.57
0
0
0.74
0.72 11,13
Sick(2)
27
231 3541
0
0.40
0.86
0.86 3,5
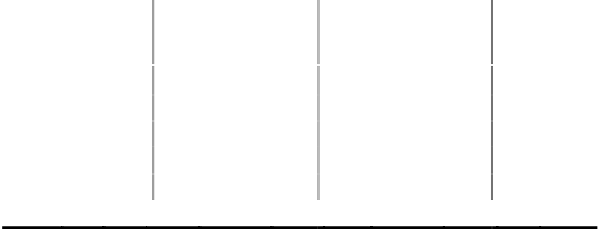










