Database Reference
In-Depth Information
Next, consider the relationship between the Temperature attribute and the Insulation rating
attribute. In our Figure 4-4 matrix, we see that the coefficient there is -0.794. In this example, the
correlation is negative, as illustrated in Figure 4-6.
Temperature
rises
Insulation
rating falls
Temperature
falls
Insulation
rating rises
Whenever attribute values move in opposite directions, the correlation is
negative
.
Figure 4-6. Illustration of negative correlations.
So correlation coefficients tell us something about the relationship between attributes, and this is
helpful, but they also tell us something about the
strength
of the correlation. As previously
mentioned, all correlations will fall between 0 and 1 or 0 and -1. The closer a correlation
coefficient is to 1 or to -1, the stronger it is. Figure 4-7 illustrates the correlation strength along the
continuum from -1 to 1.
-1 0 1
-1 ← -0.8
-0.8 ← -0.6
-0.6 ← -0.4
-0.4 ← 0
0 → 0.4
0.4 → 0.6
0.6 → 0.8
0.8 → 1.0
Very Strong
Correlation
Strong
Correlation
Some
Correlation
No
correlation
No
correlation
Some
correlation
Strong
correlation
Very strong
correlation
Figure 4-7. Correlation strengths between -1 and 1.
RapidMiner attempts to help us recognize correlation strengths through co lor coding. In the
Figure 4-4 matrix, we can see that some of the cells are tinted with shades of purple in graduated
colors, in order to more strong ly highlight those with stronger correlations. It is important to
recognize that these are only general guidelines and not hard -and-fast rules. A correlation
coefficient around .2 does show some interaction between attributes, even if it is not statistic ally
significant. This should be kept in mind as we proceed to…























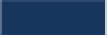






























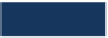
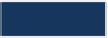














