Database Reference
In-Depth Information
confidence percentages for each of our four target sports. If RapidMiner had found some
significant possibility that an observation might have more than one possible Prime_Sport, it
would have calculated the percent probability that the person represented by an observation would
succeed in one sport and in the others. For example, if an observation yielded a statistical
possibility that the Prime_Sport for a person could have been any of the four, but Baseball was the
strongest statistically, the confidence attributes on that observation might be: confidence(Football):
8%; confidence(Baseball): 69%; confidence(Hockey): 12%; confidence(Basketball): 11%. In some
predictive data mining models (including some later in this text), your data
will
yield partial
confidence percentages such as this. This phenomenon did not occur however in the data sets we
used for this chapter's example. This is most likely explained by the fact discussed earlier in the
chapter: all athletes will display some measure of aptitude in many sports, and so their battery test
scores will likely be varied across the specializations. In statistical language, this is often referred to
as
heterogeneity
.
Not finding confidence percentages does not mean that our experiment has been a failure
however. The fifth new attribute, generated by RapidMiner when we applied our LDA model to
our scoring data, is the prediction of Prime_Sport for each of our 1,767 boys. Click on the Data
View radio button, and you will see that RapidMiner has applied our discriminant analysis model to
our scoring data, resulting in a predicted Prime_Sport for each boy based on the specialization
sport of previous academy attendees (Figure 7-17).
Figure 7-17. Prime_Sport predictions for each boy in the scoring data set.
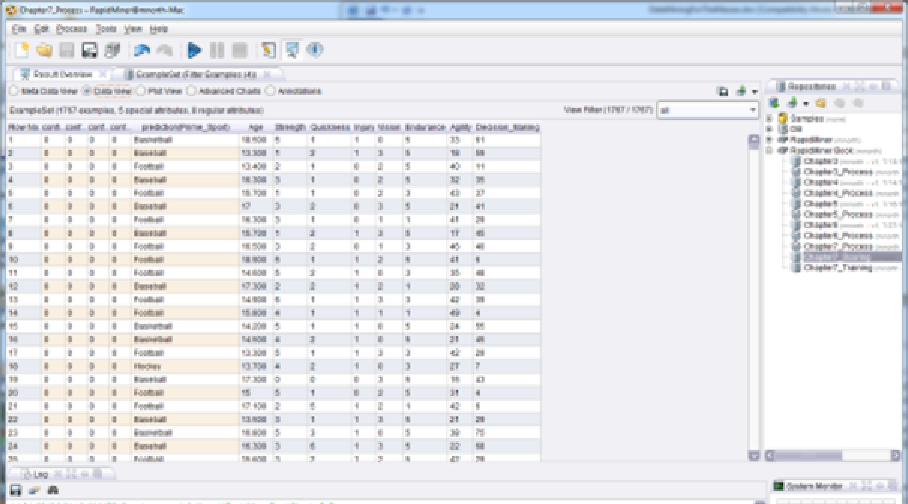
