Biomedical Engineering Reference
In-Depth Information
Table 1. Low concentrations dataset
Juice Concentration
Juice Concentration
2%
2%
4%
4%
6%
6%
8%
8%
10%
10%
16%
16%
20%
20%
Total
Total
Training
Training
19
19
17
17
16
16
22
22
21
21
20
20
19
19
134
134
Validation
Validation
1
1
1
1
13
13
6
6
6
6
6
6
6
6
39
39
Commercial
Commercial
0
0
0
0
0
0
0
0
1
1
1
1
0
0
2
2
Table 2. High concentrations dataset
Juice Concentration
Juice Concentration
20%
20%
25%
25%
50%
50%
70%
70%
100%
100%
Total
Total
Training
Training
20
20
19
19
16
16
14
14
7
7
86
86
Validation
Validation
6
6
18
18
13
13
1
1
6
6
44
44
Commercial
Commercial
0
0
2
2
0
0
0
0
19
19
21
21
Table 3. Classification with ANNs using all the variables: percent accuracy
Training (134)
134 (100%)
Training (134)
134 (100%)
Validation (39)
35 (89.74%)
Validation (39)
35 (89.74%)
Comercial (2)
2 (100%)
Comercial (2)
2 (100%)
Low Concentrations
Low Concentrations
ANN Configuration for low concentrations
Topology: 176 / 50 / 80 / 5
ANN Configuration for low concentrations
Topology: 176 / 50 / 80 / 5
learning rate: 0.0005
stop criterion: mse=5 or epochs=500.000
learning rate: 0.0005
stop criterion: mse=5 or epochs=500.000
Training (86)
86 (100%)
Training (86)
86 (100%)
Validation (44)
43 (97.72%)
Validation (44)
43 (97.72%)
Commercial (21)
21 (100%)
Commercial (21)
21 (100%)
High Concentrations
High Concentrations
ANN Configuration
Topology: 176 / 8 / 5 / 5
learning rate: 0.0005 stop criterion: mse=1 or epochs=500.000
ANN Configuration
Topology: 176 / 8 / 5 / 5
learning rate: 0.0005 stop criterion: mse=1 or epochs=500.000
The goal is to determine which solutions,
among those provided by the GA, represent good
starting points. Therefore, it would be enough to
extend the ANN learning up to the point where
it starts to converge. For this particular problem,
convergence started after 800 cycles; in order to
warrant that this step is reached, 1000 iterations
were fixed.
The selected variables were later used as inputs
of an external ANN responsible of providing the
final classification results.
Pruned Search
This approach starts by considering all variables
from groups where are gradually discarded. The
GA will steadily reduce the amount of variables
that characterise the objects from the original
data set, until getting an optimal subset that might
enable a satisfactory general classification. The
remaining set of variables is used to classify the
samples, and the results are used to determine how
relevant the discarded wave numbers were for the

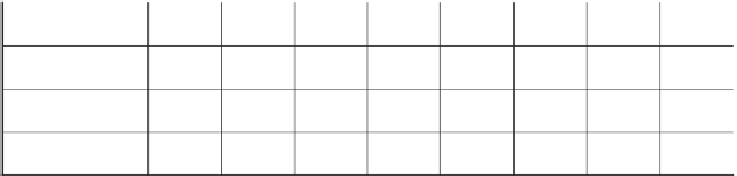








Search WWH ::

Custom Search