Database Reference
In-Depth Information
1
S
3
0.8
S
2
0.6
S
1
0.4
0.2
0
1 9 7 5 3 1
quota size
Random Classifier
Optimum Classifier
Examined Classifier
Fig. 4.7
A qualitative representation of PEM.
between the optimum model Qrecall curve and the random model (linear)
Qrecall curve, then it reaches the extent to which the potential is extracted,
independently of the number of instances in the dataset.
Formally, the PEM measure is calculated as:
PEM
=
S
1
−
S
2
,
(4.20)
S
3
where
S
1
is the area delimited by the Qrecall curve of the examined model
above the Qrecall curve of a random model;
S
2
is the area delimited by the
Qrecall curve of the examined model under the Qrecall curve of a random
model; and
S
3
is the area delimited by the optimal Qrecall curve and the
curve of the random model. The division in
S
3
is required in order to
normalize the measure, thus datasets of different size can be compared. In
this way, if the model is optimal, then PEM gets the value 1. If the model
is as good as a random choice, then the PEM gets the value 0. If it gives
the worst possible result (that is to say, it puts the positive samples at the
bottom of the list), then its PEM is
−
1. Based on the notations defined
above, the PEM can be formulated as:
Qrecall
(
j
)
n
n
j
−
S
1
−
S
2
j
=1
PEM
=
=
(4.21)
n
+
+
n
−
−
n
S
3
n
+
n
j
j
=1
j
=1
j
=1
n
j
=1
n
−
(
n
+1)
2
−
(
n
+1)
2
(
Qrecall
(
j
))
(
Qrecall
(
j
))
=
=
,
(4.22)
(
n
+
+1)
2
+
n
−
−
(
n
+1)
2
n
−
2














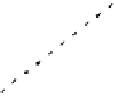
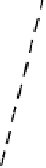
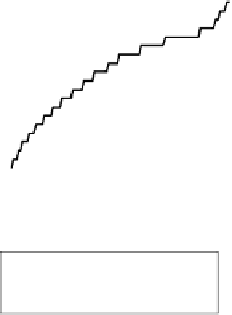





















Search WWH ::

Custom Search