Environmental Engineering Reference
In-Depth Information
illustration 9: random field modeling and updating of friction angle
We show the random field modeling approach to the friction angle presented earlier, and
its updating. We recall that the variability of the friction angle φ was assumed to follow a
normal distribution with uncertain mean μ
φ
and fixed standard deviation σ
φ
= 3°. A priori,
the mean μ
φ
was modeled through a normal distribution with parameters
28 and
µ
µ
′ =°
σ
µ
23. (see Illustration 1). This can be translated into an equivalent random ield model
for φ. Such a random field model additionally requires that a correlation function represent-
ing the spatial fluctuation of φ is prescribed. We assume a homogeneous random field with
an exponential correlation model with correlation length 2 m:
′ =
°
∆
z
rz
ϕ
∆
() exp
=
−
,
(5.36)
2m
where Δ
z
= |
z
1
−
z
2
| is the distance between two points and
r
φ
is the correlation coefficient
between φ at these points. The marginal distribution must be equal to the prior predictive
distribution (analog
Equation 5.35
)
, which is the normal distribution with mean 28° and
standard deviation
(.
234
°+°= ° The covariance function of the random field is
)
2
(
3
)
2
38
.
.
zz
−
[
]
=
1
2
Cov
ϕϕ
(),()
z
z
( .
234
° +
)
2
exp
−
(
3
°
).
2
(5.37)
1
2
2
m
We note that, due the uncertainty in the mean, the covariance becomes
(. ) as
Δ
z
→ ∞. Since the marginal is the normal distribution, it is consistent (and convenient) to
model the random field {φ} as a normal (Gaussian) random field.
For illustration purposes, we model only a one-dimensional random field {φ} in the
domain
z
∈ [−10,10m]. We assume that the three measurements considered in Illustration 1
(φ
1
= 25.6°, φ
2
= 25.5°, φ
3
= 24°) are taken at locations
z
1
= −2
m
,
z
2
= 0m, and
z
3
= 2m. The
likelihood function describing these measurements is given according to Section 5.3.3 as
σ
µ
2
′ =
234
°
2
3
∏
1
L
({
ϕ
})
=
f
(
ϕ
−
ϕ
(
z
)).
(5.38)
ε
i
i
i
=
For simplicity, we assume that the measurements are perfect (this was also implicitly
assumed in the previous illustrations). In this case,
f
ε
is the Dirac delta function δ.
Since the random field is Gaussian, its posterior is also Gaussian and an analytical solu-
tion is available for updating it with the observations (e.g., Straub 2012). The resulting pos-
terior mean and standard deviation is shown in
Figure 5.7.
The posterior random field is no longer homogeneous. The posterior uncertainty is smallest
at the locations where the samples are taken and largest at the points furthest away from the
sample locations. Here, because of the assumption of no measurement error, the posterior
standard deviation at the location of the samples is zero. At a distance Δ
z
→ ∞ from the mea-
surements, the posterior mean is 26.36° and the posterior standard deviation is 3.39°. These
values are slightly higher than those obtained earlier for the predictive posterior distribu-
tion calculated in Illustration 8. The reason for this difference is that the prior random field
model accounts for the correlation among the friction angle values at the measured loca-
tions, φ(
z
1
), ϕ(
z
2
), and φ(
z
3
) due to their proximity. This correlation reduces the information
content of the samples, similar to a correlation among measurement errors. If we considered
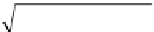


Search WWH ::

Custom Search