Information Technology Reference
In-Depth Information
Table 13.2
Classification accuracy (in percent) of k-NN, Naive Bayes, AdaBoost, and multiclass
SVMontheUCSDdataset
Method
Trial-1
Trial-2
Trial-3
Trial-4
Average
multiclass SVM
96.83
90.63
96.88
95.24
94.90
k-NN
93.65
87.5
93.75
92.06
91.74
Naive Bayes
71.43
79.69
75.00
77.78
75.98
AdaBoost
73.02
70.31
73.44
73.02
72.45
0.9
Light
0.8
0.7
0.6
Medium
0.5
0.4
0.3
0.2
Heavy
0.1
Light
Medium
Heavy
Fig. 13.5
Confusion matrix of the classification results of our method on the UCSD traffic highway
dataset (Mean accuracy: 94.90 %)
Table 13.3
Classification accuracy (in percent) of our method, Sobral et al. [
24
], Chan and
Vasconcelos [
5
] on the UCSD dataset
Method
Trial-1
Trial-2
Trial-3
Trial-4
Average
Chan et al. [
5
]
N/A
N/A
N/A
N/A
95.00
Our method
96.83
90.63
96.88
95.24
94.90
Sobral et al. [
24
]
95.20
95.30
93.80
93.70
94.50
features for the representation of video segments to be able to better discriminate
between
medium
and
heavy
traffic.
Table
13.3
provides a comparison of our approach with the solutions developed
by Sobral et al. [
24
], Chan and Vasconcelos [
5
] in terms of classification accuracy.
Since we adopted the same evaluation strategy as [
5
,
24
], the results are directly
comparable. Our method which uses dense optical flow-based motion descriptors and
multiclass SVM demonstrates comparable performance and very promising results
on the UCSD dataset. In addition to its classification accuracy, our method processes
and analyzes 15 frames per second on average, without using any special purpose
hardware. This makes our approach suitable for real-time video analysis.

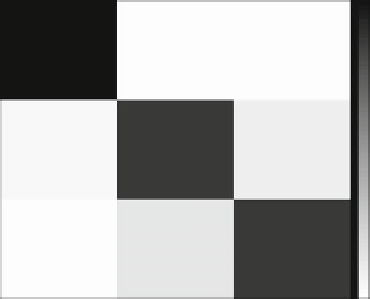
































































































Search WWH ::

Custom Search